>>> Proteins, proteins everywhere…
Proteins are the employees of the cell, working to maintain its survival, where their specific function is determined by their structural shape derived from instructions coming from the amino acid (AA) sequence encoded in our genes. For example, antibody proteins are Y-shaped, and this similitude to hooks allows them to hook to pathogens ( i.e. viruses, bacteria) and detect and tag them for extermination.
To understand how these employees go from AA sequence to their energy efficient 3D structure, the following video will be helpful. In summary, biochemists use 4 distinct aspects to describe a protein structure: A primary structure which consist of AA sequence, a secondary structure consisting of repeating local structures from this AA sequence held together by chemical bonds called hydrogen bonding forming α-helix, β-sheet and turns that are local, a tertiary structure consisting of the overall shape of a single polypeptide chain (long AA chain) by non-local chemical interactions, and a possibly quaternary structure if the protein is made by more than one polypeptide chain.
Elucidating the shape of the protein is an important scientific challenge because diseases like diabetes, Alzheimer’s, and cystic fibrosis arise by the misfolding of specific protein structures. The protein folding problem is to try and solve the right protein structure amidst many structural possibilities. A knowledge of protein structure will allow to combat deadly human diseases and use this knowledge within biotechnology to produce new proteins with functions such as plastic degradation.
Currently, the accurate experimental methods to determine the protein shape rely on laborious, lengthy, and costly processes (Figure 1). Therefore, biologists are turning to AI to help diminish these factors and speed up scientific discoveries with the potential of saving lives and bettering the environment.

“The success of our first foray into protein folding is indicative of how machine learning systems can integrate diverse sources of information to help scientists come up with creative solutions to complex problems at speed”
These were the words of Google’s AI DeepMind developers after project AlphaFold, which aims to use machine learning to predict 3D protein structure solely from amino acid sequence (from scratch), won the biennial global Community Wide Experiment on the Critical Assessment of Techniques for Protein Structure Prediction (CASP) competition on 2018. CASP is used as a gold standard for assessing new methods for protein structure prediction, and AlphaFold showed “unprecedented progress” by accurately predicting 25 out of the 43 proteins in the set (proteins which 3D structures had been obtained by conventional experimental means but not made public), compared to the second team only predicting 3 out of the 43 proteins.
Deep learning efforts attempting to do what AlphaFold have focused on secondary structure predictions using recurrent neural networks that does not predict the tertiary and/or quaternary structure needed to for the 3D protein shape due to the complexity of predicting the tertiary structure from scratch.
AlphaFold is composed of deep neural networks trained to 1) predict protein properties, namely the distances between AA and angles made by chemical bonds connecting these AA, and 2) predict the distances between every pair of protein residues and combine the probabilities into a score and use gradient descent, a mathematical method used widely in machine learning to make small incremental improvements, to estimate the most accurate prediction (Figure 2).
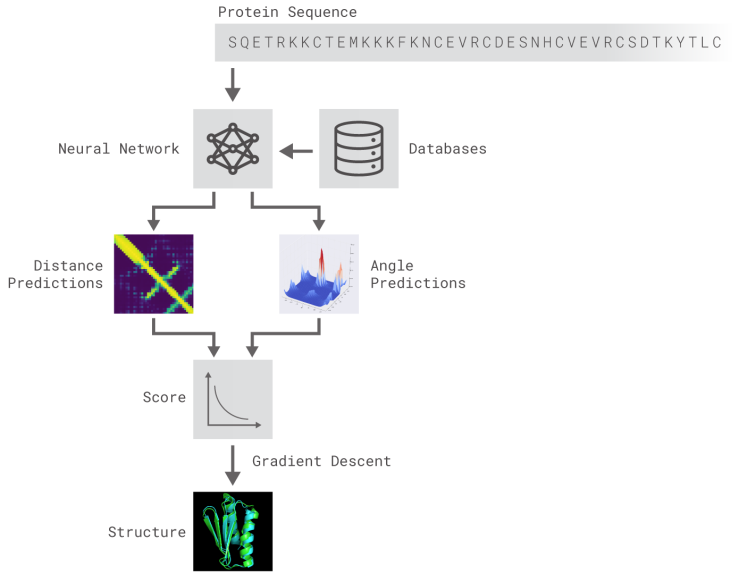
Even though there is much more work to do for a precise accurate AI use to try and solve the protein folding problem and speed up solutions to some of our world’s most grave problems, AlphaFold is undoubtedly a step in the right direction.